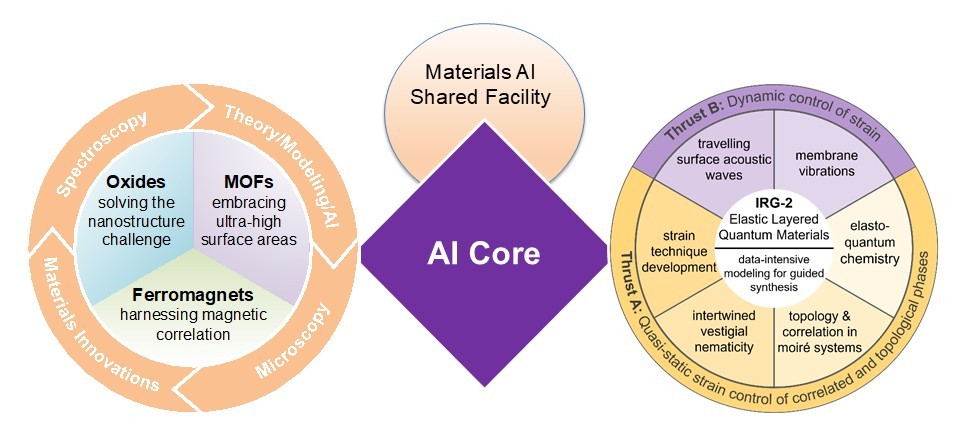
MEM-C is focused on AI-driven materials discovery with the MEM-C AI Core. AI Core faculty include Ting Cao, Scott Dunham, Lilo Pozzo and Xiaosong Li. MEM-Seed faculty have expanded this group to include Kevin Jamieson and Shijing Sun. The AI Core faculty and students provide MEM-C experimental groups with research assistance and are embedded directly in IRGs, ensuring deep integration with the Center’s research activities. Additionally, MEM-C has increased computing power of the Center’s Shared Facilities (MEM-C Hyak nodes) to provide research infrastructure to support this work.
Focus areas of MEM-C AI Core’s research includes:
- Cao’s work integrating machine learning techniques with first-principles calculations, and collaborations with condensed matter theory (D. Xiao) and experiments (Chu, Xu, Yankowitz, Yang), has uncovered novel insights into how atomic-scale interactions influence emergent electronic properties in these systems. Accurately modeling the structural relaxation within moiré superlattices is essential for understanding their electronic behavior. The Cao group is employing machine learning force fields trained on density functional theory (DFT) calculations to perform large-scale structural relaxations of twisted bilayer TMDs across various twist angles.
- Pozzo’s work expands MEM-C’s AI Core mission to serve the Center and the broader community by advancing AI-driven experimentation and analysis for acceleration of materials research. The Pozzo group works to advance the development of self-driving laboratory platforms integrating the MEM-C shared facilities’ small-angle X-ray scattering (SAXS) for structure analysis.
- Jamieson’s AI Core research advances data selection techniques for large-scale visual-language model pretraining, a problem commonly encountered in web-curated datasets but broadly applicable across domains. The Jamieson group is working to introduce novel methods for assessing data quality that improve the selection of pretraining data, ultimately enhancing model performance on downstream tasks. Although work focuses on image and text modalities from web data, the underlying principles are directly relevant to materials science, where leveraging historical multi-modal experimental data for pretraining or fine-tuning could significantly improve predictive modeling and discovery.
- New collaborative MEM-Seed work by Jamieson and Sun is developing an AI-driven robotic synthesis platform for metal-organic frameworks (MOFs)